Track all of your key business metrics from one screen
GET STARTED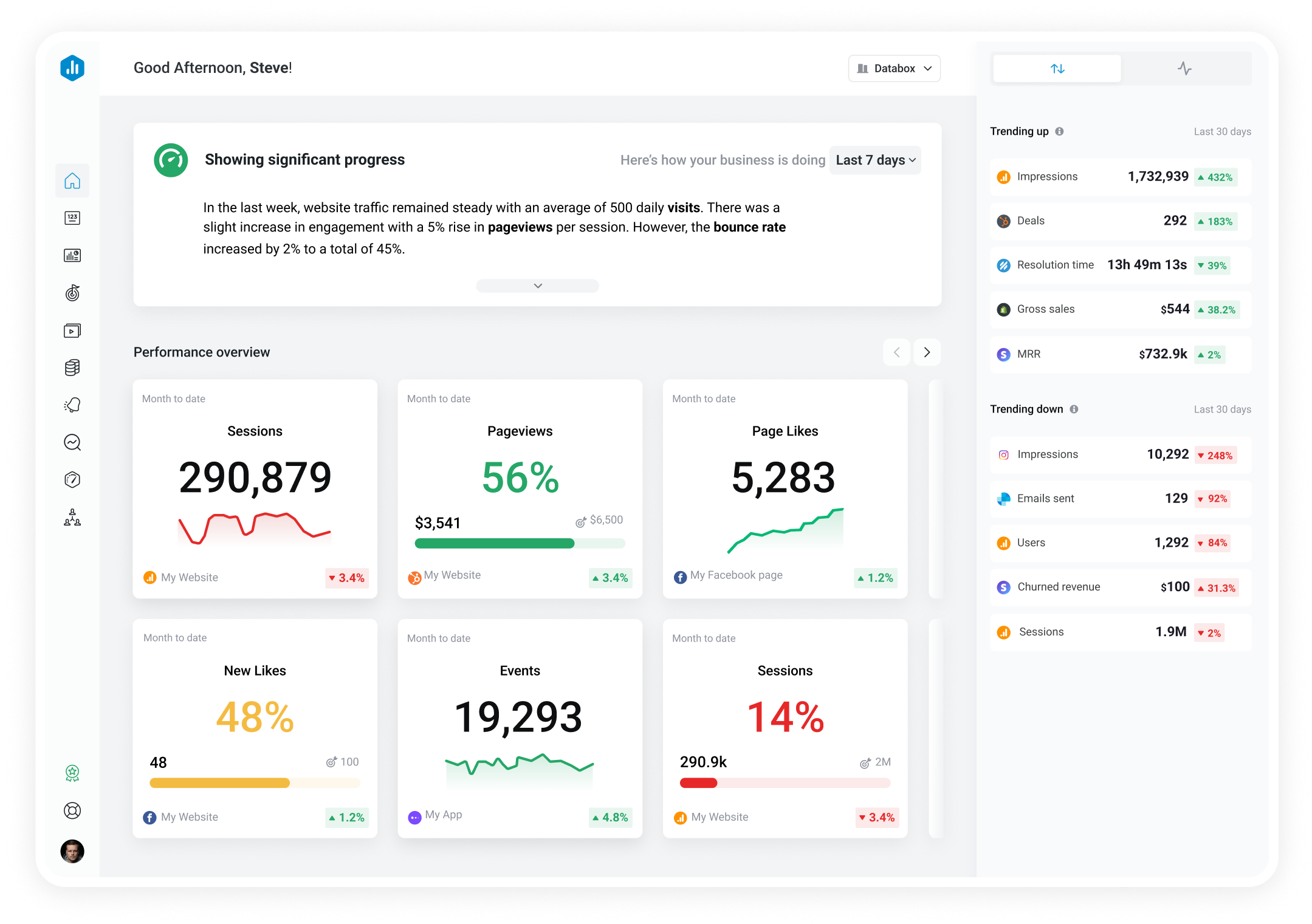
The Average Selling Price metric represents the average price at which a product or service is sold over a defined period of time. It is calculated by dividing the total revenue generated by the number of units sold.
With Databox you can track all your metrics from various data sources in one place.
The Average Selling Price (ASP) is a financial metric companies use to determine the average price at which they sell their products or services.
By tracking the average selling price over time, businesses can identify trends in pricing, monitor changes in customer behavior, and assess the overall health of their sales operations.
It is important to note that the metric can vary across different products or services within a company’s portfolio.
To calculate your average selling price, you first need to determine the total revenue you generated from sales and identify the number of units you sold.
When you have this data, you can use the following formula:
Average Selling Price (ASP) = Total Revenue / Number of Units Sold
Let’s say a company generated total revenue of $500,000 from the sales of a specific product line. During that period, they sold 5,000 units of the product.
Using the formula, we find that the company’s average selling price for that product line is $100.
What constitutes a “good” average selling price can vary significantly based on the context and objectives of the company.
It is crucial to consider a combination of factors like industry, price sensitivity, customer perception, value proposition, and similar ones.
For example, in the retail industry, the average selling price can vary significantly depending on the product category.
As a rough guideline, low-priced consumer goods might have an ASP range of $10 to $50, while mid-range products could be around $50 to $200. Higher-end or luxury products may have significantly higher average selling prices.
In the real estate sector, ASPs are typically associated with properties such as residential homes or commercial buildings.
However, these prices vary significantly based on location, property size, amenities, and market conditions. The average selling price for residential properties can range from tens of thousands to millions of dollars or more.
It’s essential to conduct industry-specific research and analyze competitor companies to gain a more accurate understanding of average selling price benchmarks for your particular market segment.
The average selling price of your products and services is directly tied to your company’s revenue and profitability.
And not only can it increase your bottom line, but having a higher average selling price also helps you better allocate resources towards product development, marketing, and customer service.
Here are some great strategies you can implement to increase your average selling price:
More resources to help you improve:
Used to show a simple Metric or to draw attention to one key number.
Used to illustrate numerical proportions through the size of the slices.
Used to show comparisons between values.
Databox is a business analytics software that allows you to track and visualize your most important metrics from any data source in one centralized platform.
To track Average Selling Price using Databox, follow these steps:
Adobe Analytics dashboard template uses visits, conversion rates and customer loyalty to help you understand your customers as people — what they want, need, and believe.
To calculate the average selling price per unit, divide the total revenue generated from sales by the total number of units sold.
The formula is:
Average Selling Price (ASP) per unit = Total Revenue / Total Units Sold.
To calculate the average stock price after selling, you need to consider the purchase price, quantity, and selling price of the stock.
Subtract the selling price from the purchase price for each stock trade, and multiply the result by the quantity. Then, add up these values for all trades and divide by the total quantity of stocks sold to obtain the average stock price.
The average selling price is important because it helps companies evaluate the effectiveness of pricing decisions, monitor changes in customer behavior and preferences, and assess overall sales performance.